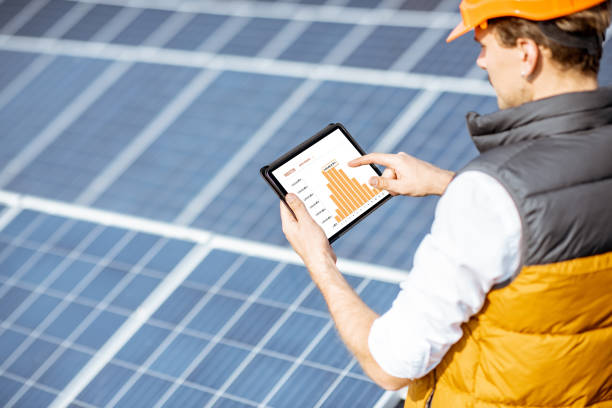
Machine learning-based technology to forecast solar power generation
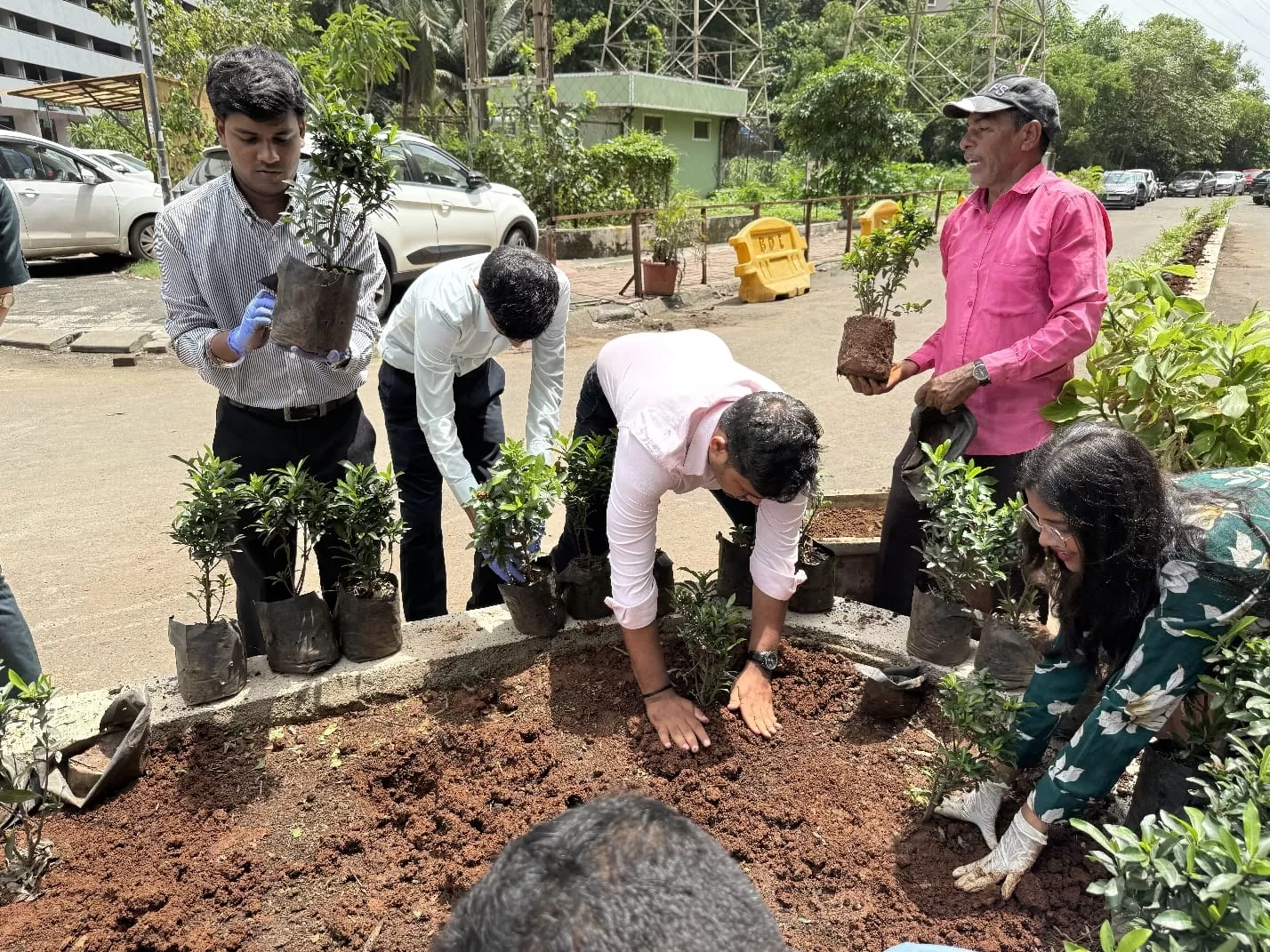
Ajmera Realty launches tree drive on Environment Day
Ajmera Realty & Infra India marked World Environment Day with a large-scale tree plantation initiative—Plant-with-Purpose—across its projects in Mumbai and Bangalore. The drive was inaugurated at Ajmera Manhattan and Ajmera Greenfinity in Wadala, with senior company officials and residents in attendance. The campaign encourages residents to embrace eco-conscious, self-reliant lifestyles by growing useful plants and trees within their communities. Horticulture expert Devendra Bhekar guided residents on creating and maintaining green spaces. Ajmera Realty planted over 500 trees..
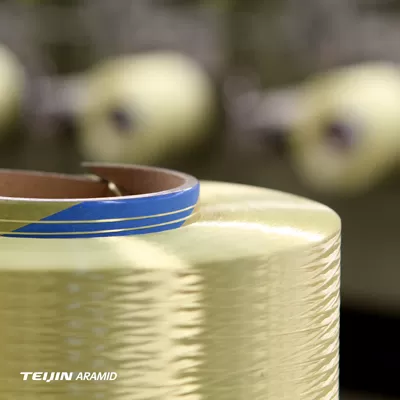
Twaron®-reinforced tyre powers Brunel’s solar race car
Teijin Aramid’s Twaron® with circular content will debut in Bridgestone’s race tyres for the 2025 Bridgestone World Solar Challenge, supporting the Brunel Solar Team’s Nuna 13 car. This marks the first use of the recycled-content aramid in a high-performance race tyre. The Twaron®-reinforced belts help enhance durability, reduce rolling resistance, and maintain lightweight strength—critical for the 3,000-km solar race across Australia. Bridgestone combines this with ENLITENTM tech and other recycled inputs to maximise environmental and performance outcomes. Teijin Aramid, a..
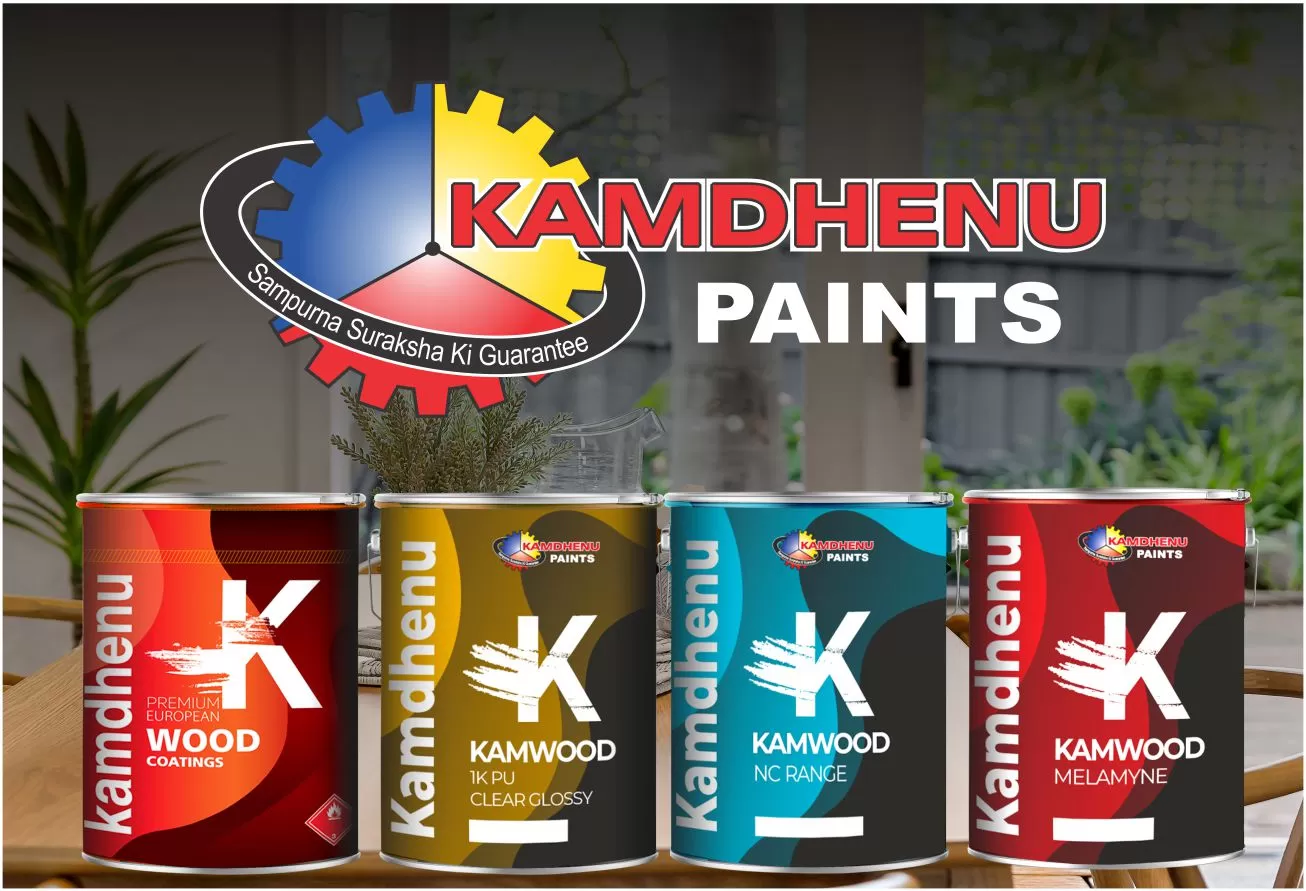
Kamdhenu Paints launches new wood coating range
Kamdhenu Paints has launched a comprehensive premium wood coating range designed for both interior and exterior applications. The collection includes high-performance solutions like Kamwood 2K PU for a rich matt or high-gloss finish, Kamwood 1K PU for clarity and stain protection, and the Kamwood Melamyne system for a smooth, durable finish. Also featured are Kamwood Wood Stains, which enhance wood grains with vibrant colour, and NC Sanding Sealer for high-build grain filling. The range is supported by Kamwood Thinners for ease of application and optimal finish. Saurabh Agarwal, MD, ..